The Impact of Machine Learning on Supply Chain Management
- andrinarose
- Feb 18
- 4 min read
Supply chain management is the backbone of modern commerce, ensuring that goods and services move efficiently from production to consumers. However, with increasing complexity, globalization, and customer expectations, traditional supply chain models often struggle to keep up. Enter Machine Learning (ML)—a transformative technology that is revolutionizing how supply chains are managed.
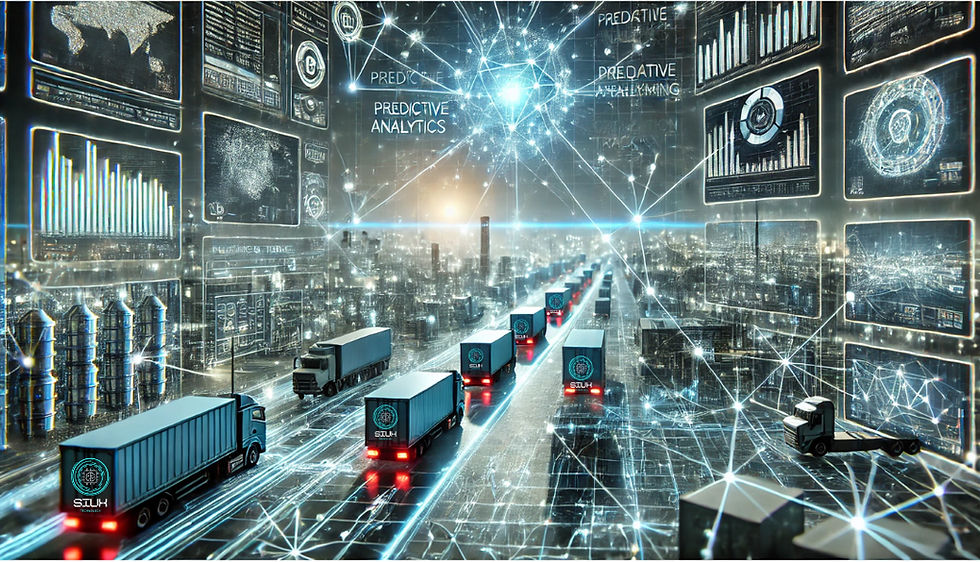
By leveraging advanced algorithms, machine learning enables businesses to optimize processes, improve decision-making, and anticipate challenges. Let’s explore how ML is reshaping supply chain management and why it’s becoming indispensable for forward-thinking organizations.
What is Machine Learning in Supply Chain Management?
Machine learning is a subset of artificial intelligence (AI) that uses data and algorithms to mimic human decision-making, improve accuracy, and provide predictive insights. In supply chain management, ML analyzes vast amounts of structured and unstructured data to uncover patterns, predict outcomes, and automate decisions.
From demand forecasting to inventory optimization, ML applications are helping businesses achieve efficiency, reduce costs, and deliver exceptional customer experiences.
Key Areas Where Machine Learning is Impacting Supply Chains
Demand Forecasting
Accurate demand forecasting is critical to supply chain efficiency. Machine learning models analyze historical sales data, seasonal trends, and external factors like weather or economic conditions to predict future demand.
Benefits:
Reduce overstocking and stockouts.
Minimize waste in perishable goods.
Optimize production schedules and inventory levels.
For example, retailers can use ML to anticipate high-demand periods and ensure shelves are stocked appropriately.
Inventory Management
Maintaining the right inventory levels is a delicate balance. ML-powered systems analyze past purchasing patterns, supplier performance, and market trends to automate inventory management.
Benefits:
Improve inventory turnover rates.
Identify slow-moving or excess stock.
Reduce holding and storage costs.
Smart warehouses use ML algorithms to dynamically allocate storage space and predict inventory replenishment needs in real-time.
Logistics Optimization
Transportation and logistics are among the most complex aspects of supply chain management. ML algorithms optimize routes, reduce fuel consumption, and improve delivery times by analyzing traffic patterns, weather conditions, and vehicle performance.
Benefits:
Lower transportation costs.
Enhance delivery reliability and customer satisfaction.
Reduce carbon emissions through optimized routing.
For example, logistics giants like UPS use ML to create dynamic routing systems that improve delivery efficiency.
Supplier Risk Management
Supplier reliability is crucial for maintaining smooth operations. ML tools analyze supplier performance metrics, market trends, and geopolitical factors to assess risks and recommend contingency plans.
Benefits:
Proactively identify and mitigate risks.
Improve supplier selection and contract negotiations.
Ensure continuity in supply chain operations.
ML can even predict potential supplier disruptions due to financial instability or natural disasters.
Quality Control and Defect Detection
In manufacturing and production, ML-powered systems use computer vision and real-time monitoring to detect defects or quality issues in products.
Benefits:
Reduce waste and production delays.
Enhance product quality and customer satisfaction.
Identify root causes of defects for continuous improvement.
For example, automotive manufacturers use ML to monitor assembly lines and ensure precision in parts manufacturing.
Real-Time Supply Chain Visibility
End-to-end visibility is a top priority for modern supply chains. ML integrates data from IoT devices, sensors, and ERP systems to provide real-time updates on inventory, shipments, and production status.
Benefits:
Enable proactive decision-making.
Quickly address disruptions or bottlenecks.
Improve transparency and collaboration across stakeholders.
This enhanced visibility allows businesses to adapt quickly to changing circumstances, such as delayed shipments or sudden demand spikes.
Enhancing Customer Experience
Meeting customer expectations is a cornerstone of supply chain success. ML enables personalized experiences by analyzing customer preferences and purchase behavior.
Benefits:
Predict delivery times accurately.
Offer tailored product recommendations.
Respond swiftly to customer inquiries through AI-powered chatbots.
E-commerce companies, for example, use ML to optimize delivery times and ensure that customers receive their orders as quickly as possible.
Challenges in Adopting Machine Learning for Supply Chains
While the benefits of ML are immense, businesses may face challenges in implementation:
Data Quality: Poor or incomplete data can hinder ML models' effectiveness.
Integration Complexity: Aligning ML tools with existing supply chain systems can be complex and time-consuming.
Cost and Expertise: ML requires investment in technology and skilled professionals to develop and maintain systems.
To overcome these challenges, organizations should start small, focusing on specific pain points, and scale ML applications gradually as they gain expertise.
The Future of Machine Learning in Supply Chain Management
The role of machine learning in supply chains will only grow as technology advances. Emerging trends include:
Autonomous Supply Chains: ML will enable fully automated supply chains that self-adjust to disruptions or demand changes.
Sustainability Insights: ML will help businesses track and reduce their carbon footprint by optimizing resource usage.
Blockchain Integration: ML combined with blockchain will enhance transparency and security in supply chain transactions.
Conclusion
Machine learning is a game-changer for supply chain management, enabling businesses to move from reactive to proactive strategies. By optimizing processes, improving visibility, and reducing risks, ML helps organizations deliver value to customers while staying ahead of the competition.
At SiUX Technology, we specialize in integrating advanced machine learning solutions into supply chains to drive efficiency and innovation. Whether you’re looking to improve forecasting, streamline logistics, or enhance visibility, our experts can help you harness the power of ML to transform your supply chain operations.
Contact us today to explore how machine learning can revolutionize your supply chain and position your business for future success.
Comments